Can your Plantower PMS5003-based Air Quality Sensor Measure PM10?
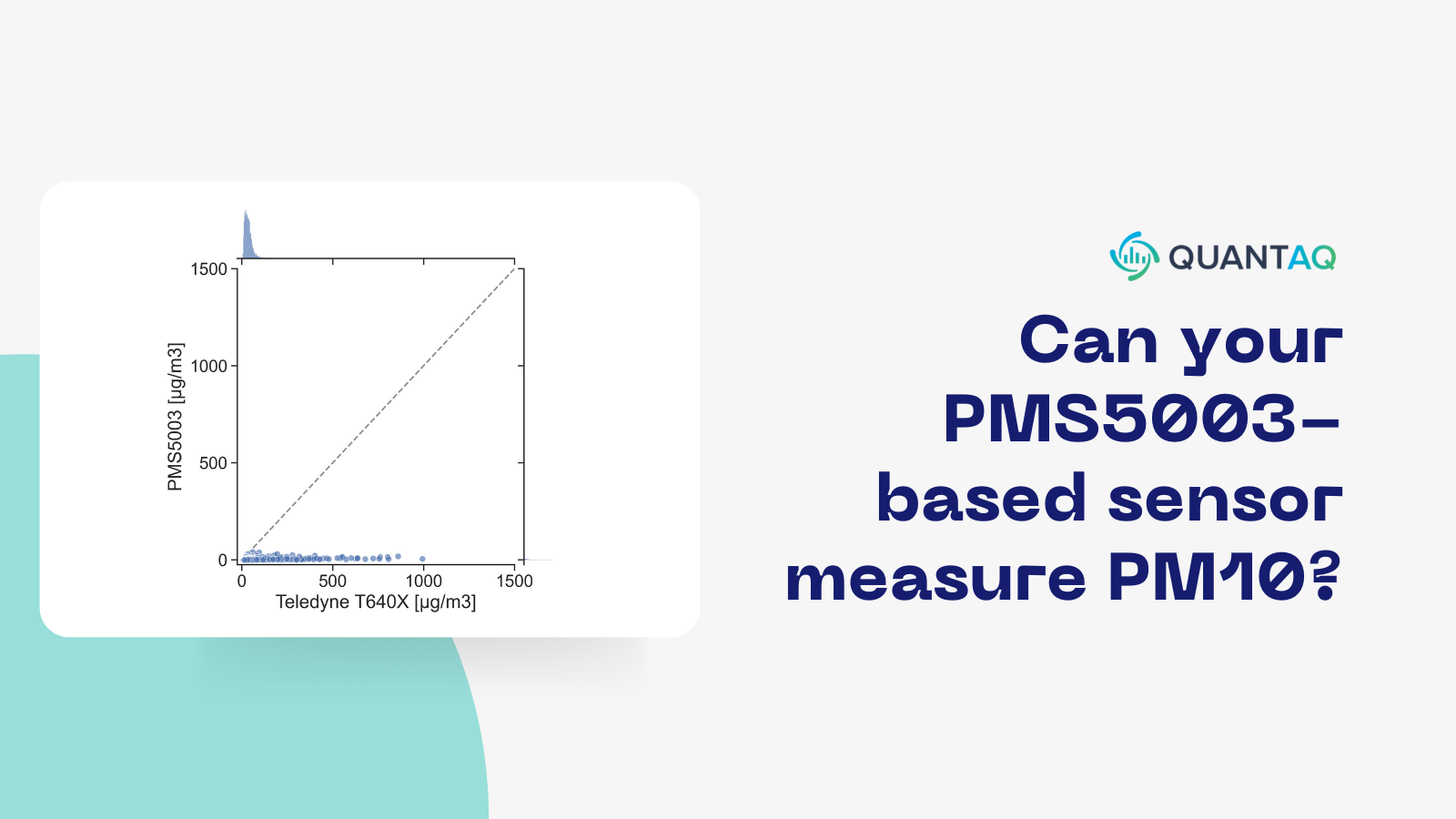
No. Let's investigate.
The Plantower PMS5003 is a low-cost particle sensor that does a (very) good job measuring the total scattered light for particles that are able to physically make it to the sensing area of the device. The peer-reviewed literature has shown the PMS5003 is unable to detect particles larger than ~1 µm and behaves most like a nephelometer [1, 2].
This doesn't mean the PMS5003 is a bad sensor; however, it's important to understand, like with any analytical instrument, what the device can and cannot measure. Nephelometers like the PMS5003 output a single scalar value that is proportional to the total scattered light of the entire aerosol distribution it measured at a point in time. In practice, this scalar value is correlated to a PM value (often, PM2.5) from a reference instrument (often an EPA FEM instrument). Unlike optical particle counters (a different low-cost particle measuring technology), nephelometers like the PMS5003 are able to measure a variety of different aerosols quite well without need for re-calibration, at least to a first degree [3]. However, the accuracy of the device is heavily reliant on the aerosol used to calibrate it. In the case of the PMS5003, it is quite accurate for background urban aerosol (relatively small particles with little to no mass contribution from large particles), but begins to falter as soon as the underlying properties of the particles you're trying to measure differ from a typical urban environment.
Let's take a look at a couple of examples.
When does it work well?
The PMS5003 does a good job estimating PM1 and PM2.5 (especially when a large fraction of the particles are < 1 µm). We can see that quite clearly in the figure below using data collected when measuring urban aerosol. Why? For two reasons:
- the urban aerosol being measured is similar enough to the calibrant (in size, optical properties, etc) that the initial calibration holds up
- there is very little contribution to the total mass from particles larger than 1 µm in this context, which limits the impact of particles between 1-2.5 µm on the PM2.5 integrated mass
This is highlighted in a number of peer-reviewed papers [4, 5] and measurements can be improved by correcting for changes in the aerosol composition and/or averaging over long periods of time to normalize the particle size distribution.
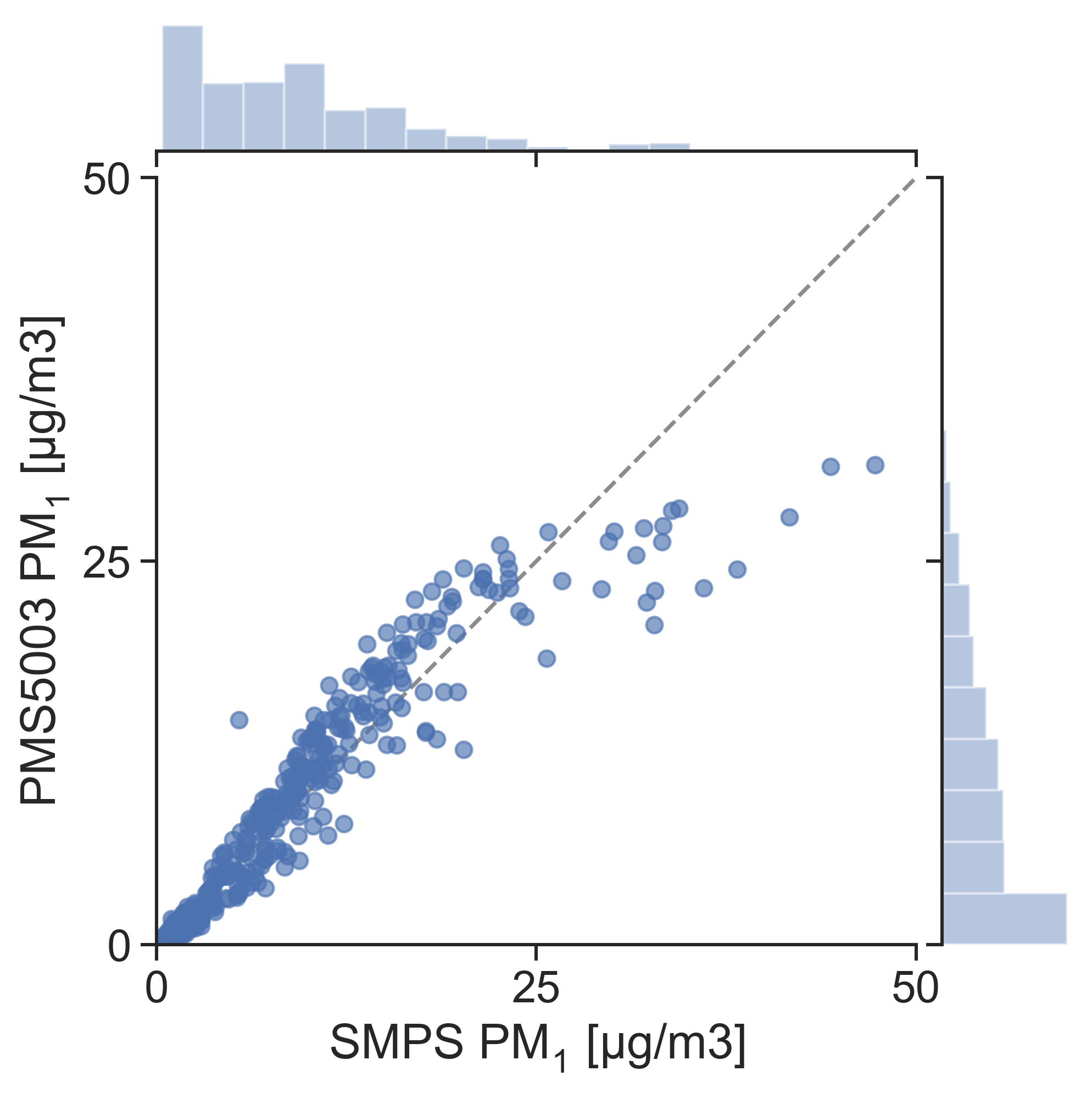
However, the same trends don't hold when large particles are present.
When does it outright fail?
Here, we take a look at some data collected in a desert environment that is impacted by frequent wind-blown dust events. The PM10 loadings often reach hourly averages of 250 µg/m3 with peaks exceeding 3,000 µg/m3!
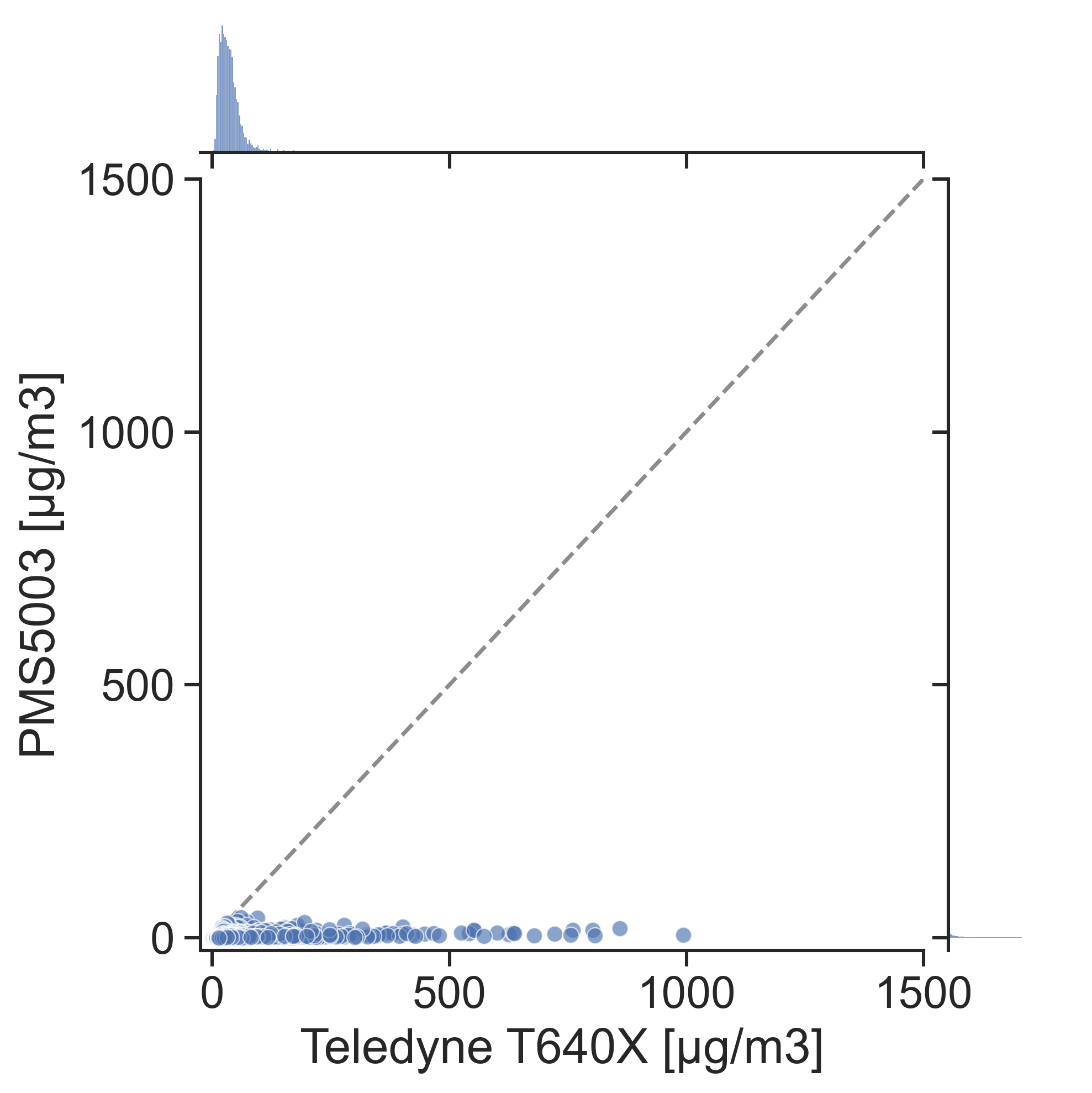
When large particles are present (i.e., PM10 >> PM2.5), the data show the inability of the PMS5003 to accurately quantify the PM10 loadings. In this case, the PMS5003 reports a 95% negative bias relative to the Teledyne T640X (an EPA FEM instrument) with a coefficient of determination that is -641.
So, why does it seem to work for some people?
There are quite a few peer-reviewed papers and other sources that report strong results (i.e., high correlation coefficient, low error) measuring PM10 with a PMS5003-based sensor. Most often, if you look at the data in these publications, you will see they are making measurements when no large particles are present - they are essentially measuring PM1, which in these instances, correlates with PM10. This should not be interpreted as the sensor being able to accurately measure PM10. Rather, it implies that in this case, PM1 and PM10 are highly correlated. If you subtract PM2.5 from PM10, you will be left with the actual large particle signal (often referred to as PMc). This value will very likely not correlate well with the reported PM10 value from the PMS5003.
An alternative way of thinking about the ability of a particle sensors ability to measure PM10 is viewing it as a classification task. Rather than ask "how strong is the correlation between this output value and a reference PM10 measurement", you could ask "how well could this sensor classify a dust event?". How would you go about doing this? It's simple. Using your reference data, find times where the PMc (PM10 - PM2.5) is significantly elevated - this will eliminate data where there is no contribution from large particles. Next, correlate your PM10 data against the reduced dataset to see if there is any correlation. If so, then your sensor is likely able to measure large particles. If not, well, it is not.
Summary
The PMS5003 can be a very useful low-cost option when used correctly. If you're trying to measure smaller particles (PM1, PM2.5) in an environment that doesn't change too much, it can be calibrated and remain quite reliable. If the environment you're making measurements in is changing, you should be careful with how you interpret the data. If you're trying to measure for dust, construction debris, or other large particles, the PMS5003 (and other low-cost nephelometers) are likely the wrong option.
Fortunately, QuantAQ has developed an alternative approach that allows you to easily measure both small and large particles simultaneously! For more information on the MODULAIR-PM, click through to read more.
For more information on making reliable, efficient air quality measurements at scale, reach out to QuantAQ at hello@quant-aq.com.
References
- Kuula, J., Mäkelä, T., Aurela, M., Teinilä, K., Varjonen, S., González, Ó., and Timonen, H.: Laboratory evaluation of particle-size selectivity of optical low-cost particulate matter sensors, Atmos. Meas. Tech., 13, 2413–2423, https://doi.org/10.5194/amt-13-2413-2020, 2020.
- Ouimette, J. R., Malm, W. C., Schichtel, B. A., Sheridan, P. J., Andrews, E., Ogren, J. A., and Arnott, W. P.: Evaluating the PurpleAir monitor as an aerosol light scattering instrument, Atmos. Meas. Tech., 15, 655–676, https://doi.org/10.5194/amt-15-655-2022, 2022.
- Hagan, D. H. and Kroll, J. H.: Assessing the accuracy of low-cost optical particle sensors using a physics-based approach, Atmos. Meas. Tech., 13, 6343–6355, https://doi.org/10.5194/amt-13-6343-2020, 2020.
- Carl Malings, Rebecca Tanzer, Aliaksei Hauryliuk, Provat K. Saha, Allen L. Robinson, Albert A. Presto & R Subramanian(2020)Fine particle mass monitoring with low-cost sensors: Corrections and long-term performance evaluation,Aerosol Science and Technology,54:2,160-174,DOI: 10.1080/02786826.2019.1623863
- Barkjohn, K. K., Gantt, B., and Clements, A. L.: Development and application of a United States-wide correction for PM2.5 data collected with the PurpleAir sensor, Atmos. Meas. Tech., 14, 4617–4637, https://doi.org/10.5194/amt-14-4617-2021, 2021.